0
23rd August 2024 8:00 am
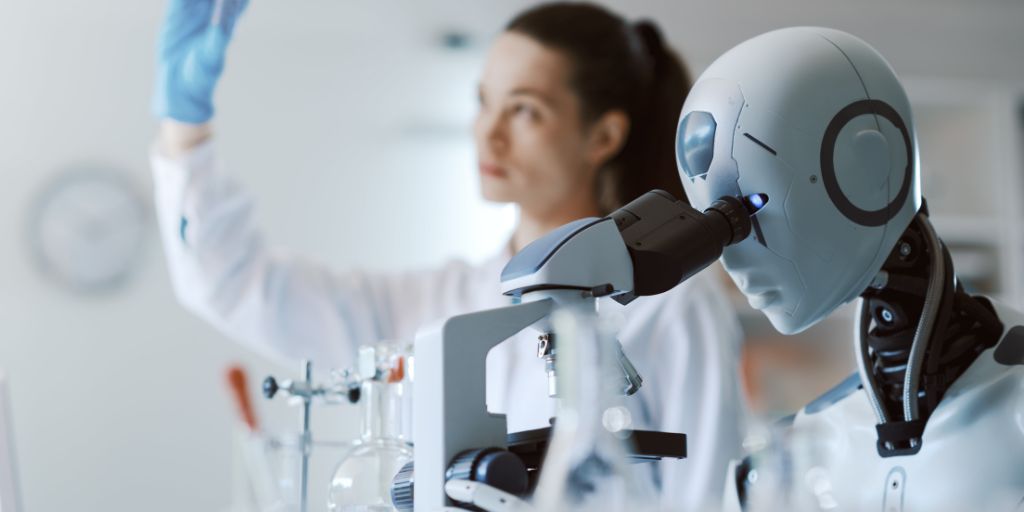
The healthcare and life sciences industries are expected to be among the biggest beneficiaries of artificial intelligence (AI). Statista estimates that the market size of AI in healthcare globally will touch a massive $187.95 billion by 2030. This bodes particularly well for Europe, which has been battling challenges such as burgeoning aging population and dwindling healthcare personnel.
The World Health Organisation (WHO) expects the population aged 60 and older to reach 247 million by 2030 and over 300 million by 2050, impacting healthcare, urban planning, housing and transport. The European Parliament estimates a shortage of 4.1 million healthcare personnel by 2030, including 0.6 million physicians, 2.3 million nurses, and 1.3 million other healthcare professionals.
AI is, therefore, ripe for use by the European healthcare system to not only overcome these challenges but also to reap the enormous potential of AI. A MedTech Europe and Deloitte study found that AI could lead to significant positive outcomes for health systems. The potential benefits of AI in healthcare not only included 400,000 lives saved annually but also 200 billion Euros of annual savings. AI can free up about 1.8 billion working hours yearly – equivalent to adding 500,000 full-time healthcare professionals.
AI in frontline clinical work
Almost every gap in the life sciences industry is an opportunity to use AI. In areas such as patient recruitment, drug discovery, drug testing and globalising trials, there is tremendous potential for AI application.
The drug development process is typically time-consuming, but AI automation can significantly accelerate the testing phase, resulting in faster releases of drugs into the market. Through AI algorithms, the analysis of data from huge groups becomes more efficient, helping to spot trends and designs. Similarly, machine learning (ML) algorithms can expedite medicine development by analysing vast amounts of genomic disease data to pinpoint possible drug targets that help forecast and enhance the effectiveness of drugs. This is coupled with AI and ML’s capability of predicting the effectiveness of potential drug treatments for particular patient populations, such as those with compromised immunity.
AI potential in data analysis in life sciences
Outside of frontline clinical work, AI can help analyse data collected from patient wearables or sensors used for in-home care or virtual hospital settings to provide early warning or predictive diagnosis of various conditions. Here, one of the game-changing uses of AI in data collection is in Electronic Health Records (EHRs). AI algorithms can help study data from vast populations to identify trends and patterns.
The use of Natural Language Processing (NLP) and predictive analysis in AI has grown so prolific that the onset of certain medical conditions in patients can now be predicted based on their personal medical history, lifestyle, family history and local environmental factors. A subset of AI and ML, predictive health analytics is being used not only for improving patient outcomes but also for early prediction of disease onset. A study discovered that a deep learning algorithm can predict Alzheimer’s disease six years before the diagnosis with an accuracy of over 90%. Such use cases can revolutionise diagnosis and treatment with enhanced patient outcomes.
The life sciences industry processes generate vast data through clinical trial recruitment or drug dissemination. Predictive analytics using AI can help analyse patient data faster and provide necessary interventions.
Similarly, the life sciences industry can deploy AI through chatbots and virtual assistants to help patients get early access to healthcare and health information, thereby reducing the wait time for doctor consultations. Another use case is processing insurance claims, where AI can speed up processes by doing away with manual and time-consuming tasks.
Transformative capabilities of Generative AI in life sciences
Generative AI can learn from the vast amounts of unstructured data in life sciences and use that learning to generate new data, such as synthetic patient data, diagnostic reports, and personalised treatment plans. This technology can help doctors to choose the most effective medicines, minimise undesirable side effects, and determine the right dosage based on the individual’s genetic profile. Also, generative AI can help physicians diagnose and treat certain conditions by producing images that mimic those conditions to help train physicians.
The use of AI in life sciences has a wide range of applications, including data extraction and analysis, which can lighten the load and speed up investigations due to the critical shortage of healthcare workers in Europe. AI-enabled data extraction and analysis can yield valuable insights that can be used to improve patient care and outcomes.
Generative AI can transform the life sciences industry by automating tedious and error-prone operational work. Clinicians can record a patient’s entire visit using the AI – from when the patient’s information is added in real-time to filling in any gaps and prompting the clinician to fill them in. They can effectively turn the dictation into a structured note with conversational language.
A word of caution
As with any disruptive innovation over the years, the benefits come with risks that must be examined and mitigated. A paper published in 2022 by the European Parliament underlined significant benefits of AI from complementing health personnel amid shortage and enhanced efficiency levels for optimum utilisation of health-related resources to improved sustainability measures and sharper policies. However, it also acknowledged several dangers of using AI in healthcare, pointing to issues such as potential errors, risk of bias, a lack of transparency, and vulnerability to hacking. The key to overcoming these challenges will be several risk assessment methods and policies to minimise the risks and maximise the benefits.
Given that the industry’s most significant data source is patient information, which is sensitive, securing the same and mitigating cyber threats will be a priority. In addition, barriers to AI adoption could include potential patient harm due to AI-induced misdiagnosis and the risk of biases being introduced in medical AI, among other things.
Nonetheless, if the recommendations by the European Parliament are anything to go by, proper risk and threat mitigation coupled with steps like developing a framework for better accountability should help the life sciences industry optimise AI safely.
Healthcare professionals implementing AI must adhere to ethical standards such as mandating algorithmic transparency and explainability, assigning liability and accountability etc. It could also include steps such as giving IT professionals and data scientists adequate clinical context, verifying that data inputs are valid, accurate and sourced correctly, and interpreting AI black-box solutions to provide patients with real-time and post-facto advice.
The advantages of incorporating AI into healthcare are evident, but it is important to emphasise that its effectiveness depends heavily on the accuracy of the data it relies on. Additionally, organisations must be diligent in eliminating cognitive bias and safeguarding patient privacy when deploying AI in healthcare. While AI will undoubtedly shape the future of healthcare, establishing proper guidelines is essential to unlock its full potential.